Effective data annotation is crucial for the success of AI and machine learning projects. However, relying on generic workflows can lead to inefficiencies and inconsistent results. Custom in-house workflows offer a solution tailored to the specific needs of your project. They can significantly improve both the quality and productivity of your data annotation efforts.
This article explores why custom workflows are essential and how they can enhance your processes. It also provides practical steps to set up your own in-house system. By optimizing workflows to align with your unique requirements, you can ensure that your annotated data meets the highest standards, driving better outcomes for your AI projects.
Generative AI is advancing rapidly, producing a wide range of content, including written, audio, and visual formats. McKinsey predicts that by the end of this decade, generative AI will match the average person’s skill level in these tasks. Additionally, by 2040, it could even compete with the highest 25% of people, much sooner than previously estimated. This rapid progress underscores the increasing demand for high-quality, annotated data, making custom in-house workflows essential for maintaining a competitive edge.
Off-the-shelf solutions often fall short when addressing the unique requirements of your projects. Custom workflows offer the flexibility to tailor processes to specific needs, leading to improved outcomes.
Here are the key reasons to consider developing custom in-house workflows:
- Specific project requirements: Generic solutions may not fully meet the demands of your projects. Custom workflows allow adjustments to align with unique data sets and quality standards, ensuring better results.
- Efficiency gains: Custom workflows can streamline the annotation process, reducing bottlenecks and speeding up project timelines.
- Consistency and quality: By standardizing processes tailored to your projects, custom workflows help maintain consistency and high quality across all annotated data.
- Scalability: Custom workflows can be designed to scale with your project, accommodating increasing data volumes without compromising quality.
Moreover, relying on generic data annotation outsourcing services might limit your ability to fully control and optimize the process. Developing in-house workflows provides the opportunity to refine and adapt as your project evolves.
To stay ahead in an environment where data annotation quality directly impacts the effectiveness of AI models, investing in custom in-house workflows is a strategic move. By doing so, you can ensure your data meets the highest standards, ultimately leading to more accurate and reliable AI outcomes.
How Custom Workflows Improve Quality and Productivity
Custom workflows offer a way to fine-tune your data annotation process. Unlike one-size-fits-all solutions, these workflows adapt to the specific requirements of your project. This adaptability can lead to significant improvements in both quality and productivity.
One of the main advantages of custom workflows is their ability to align with your project’s unique requirements. When you design a workflow around the specific data you’re working with, you ensure that every step in the process is optimized. For example, if your project involves complex image annotation, it allows you to include extra validation steps that might be unnecessary for simpler tasks. This targeted approach reduces errors and enhances the accuracy of your annotations.
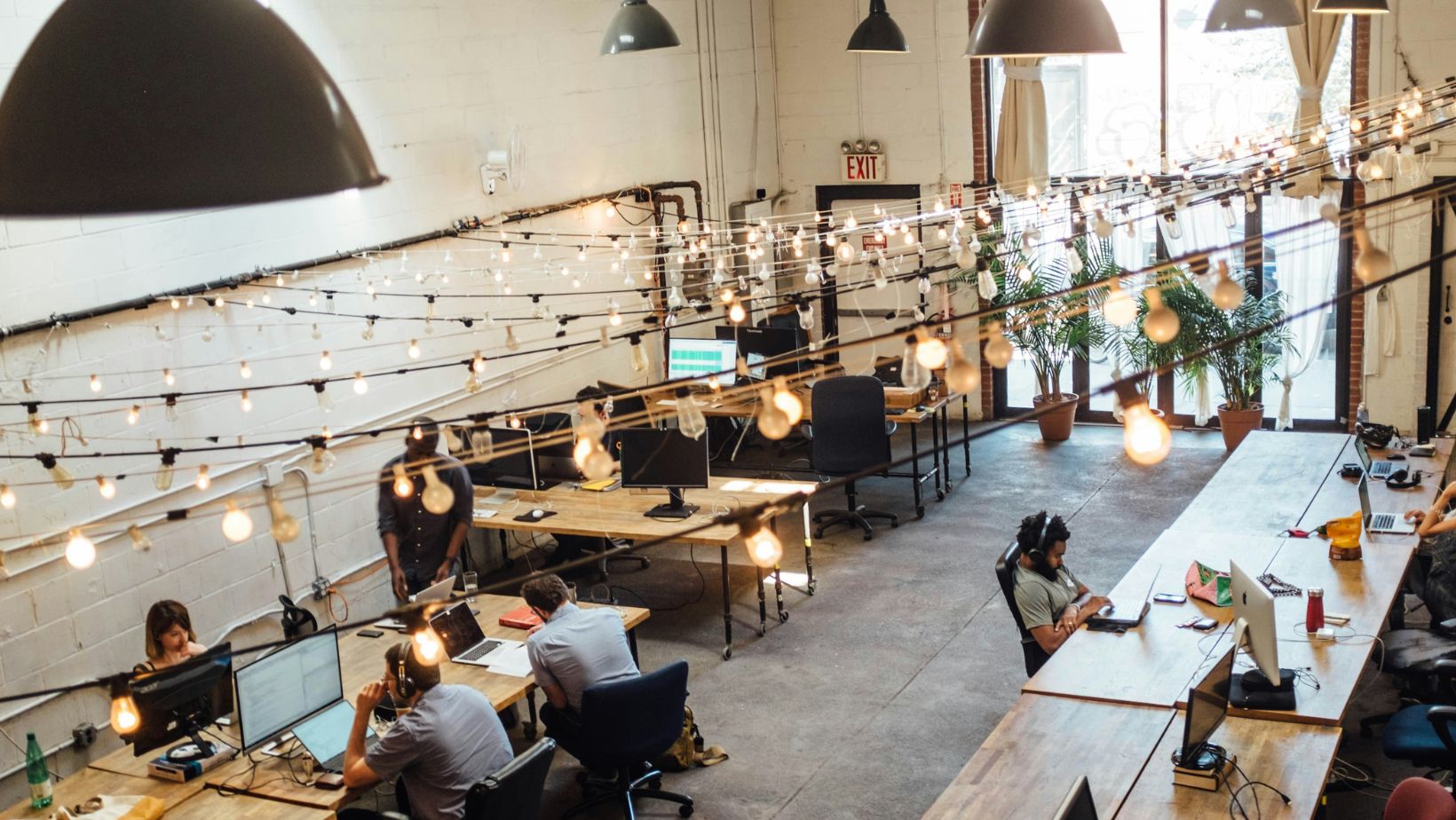
Another benefit is the ability to streamline the annotation process. It also reduces the cognitive load on annotators, allowing them to work more efficiently. When your team isn’t bogged down by irrelevant tasks, they can focus on what matters most — producing high-quality annotated data.
Custom workflows also enhance consistency across your projects. By standardizing the processes used to handle your data, you create a reliable framework that ensures uniformity. This consistency is key when working on large-scale projects, where even small variations can lead to significant discrepancies in the final output.
Here’s how custom workflows contribute to better quality and productivity:
- Tailored processes. Custom workflows allow you to curate each step, which improves accuracy and reduces errors.
- Increased efficiency. By eliminating unnecessary steps and focusing on the critical aspects of your project.
- Enhanced consistency. Standardizing processes across all projects ensures that your data is annotated in a uniform way, reducing discrepancies.
Whether you’re handling a small dataset or a massive one, a custom workflow can be adjusted to meet the demands of your project. This scalability ensures that you maintain the same level of quality and productivity, regardless of the project size.
Steps to Set Up Effective Processes
Creating an effective in-house workflow for data annotation doesn’t have to be complicated. It’s about breaking down the process into manageable steps and making adjustments along the way. Here’s a straightforward approach to get started:
-
Assess Your Current Process
Identify any bottlenecks or inefficiencies that slow down your data annotation process. Look at the tools you’re using, how tasks are assigned, and how data is managed. This assessment will help you understand what’s working and what can be improved.
-
Define Your Project Requirements
Outline what you need in terms of accuracy, speed, and output format. This step helps tailor your workflow to meet these exact needs, ensuring that nothing is overlooked.
-
Design the Workflow
This might involve creating new steps, removing unnecessary ones, or reassigning tasks to different team members. Ensure that each step in the process is clear and that everyone involved understands their role.
-
Implement and Test
Start with a small batch of data to see how it works in practice. Testing helps you spot any issues early and make adjustments before scaling up.
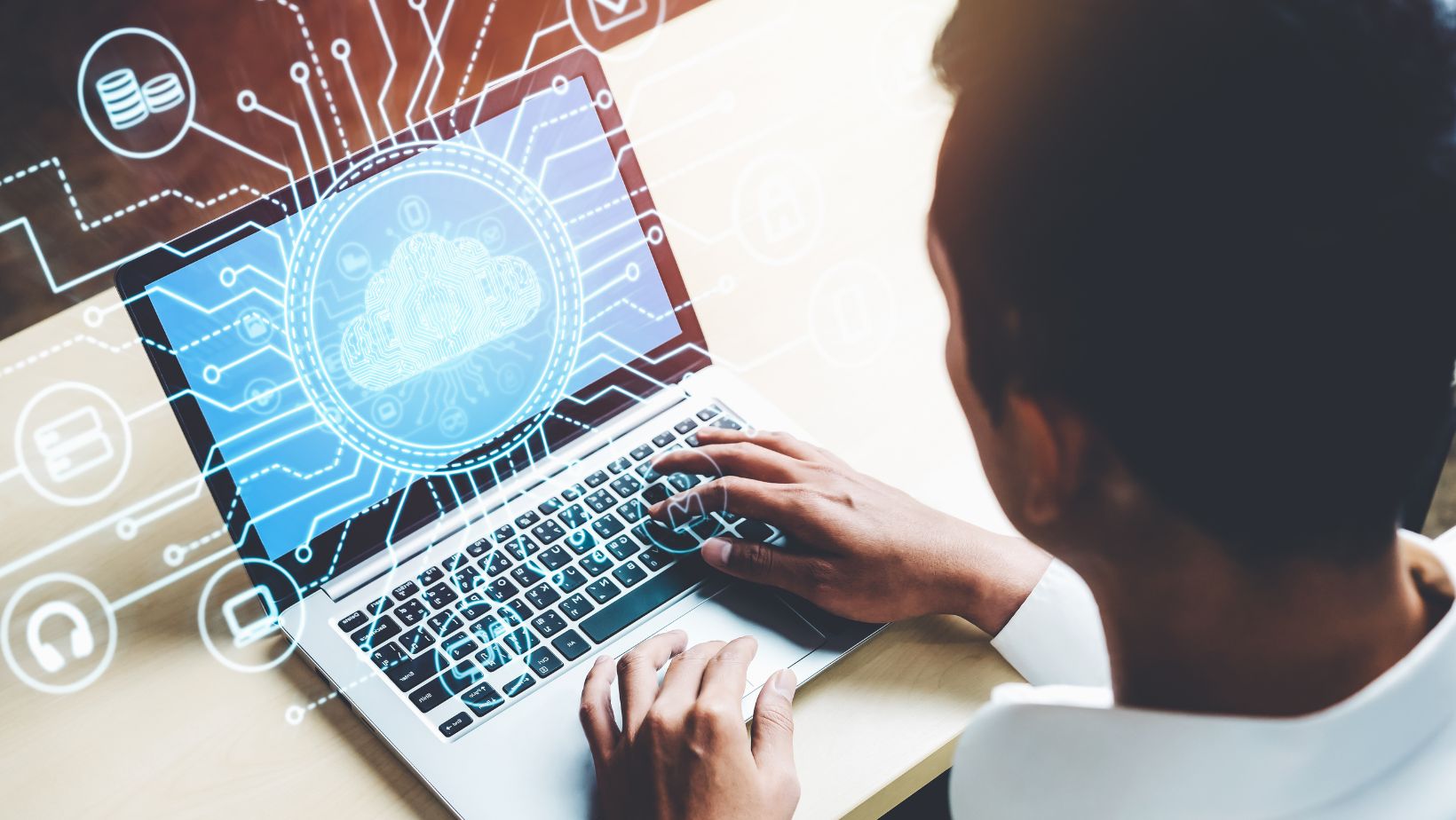
Don’t skip this step — testing ensures that your workflow is both efficient and effective.
-
Monitor and Refine
Finally, keep an eye on the workflow as your project progresses. Collect feedback from your team and look at performance metrics. Use this information to refine and improve the process.
To Sum Up
Custom in-house workflows can make a significant difference in the quality and efficiency of your data annotation efforts. Implementing abovementioned tips sets a solid foundation for future success as well.
Now is the time to evaluate your existing processes and start making the necessary adjustments. Start these best practices to create an effective, scalable practices that meet your project goals. It will position your team to handle more complex data annotation tasks with confidence and precision.
More Stories
The Benefits Of Custom Headless Cms Development Over Off-The-Shelf Solutions
How Online Bookies Are Shaping the Way We Bet on Sports in Europe
How Live Tournament Poker Returned Stronger After Pandemic Disruptions in 2022